透過對大氣環境中的空氣質量進行預測和模擬,了解澳門空氣質量每日變化的規律,影響因素與機制。
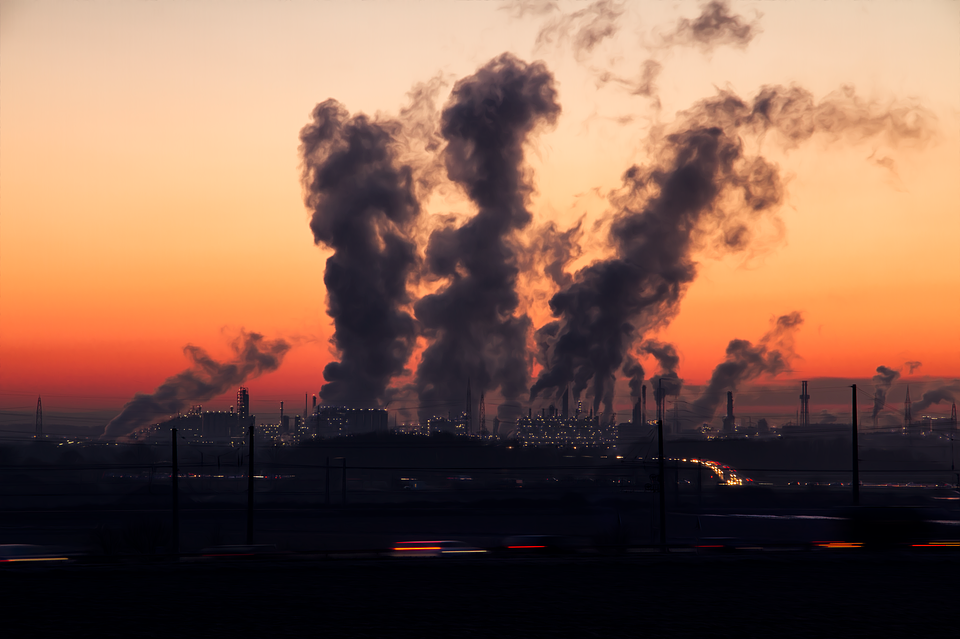
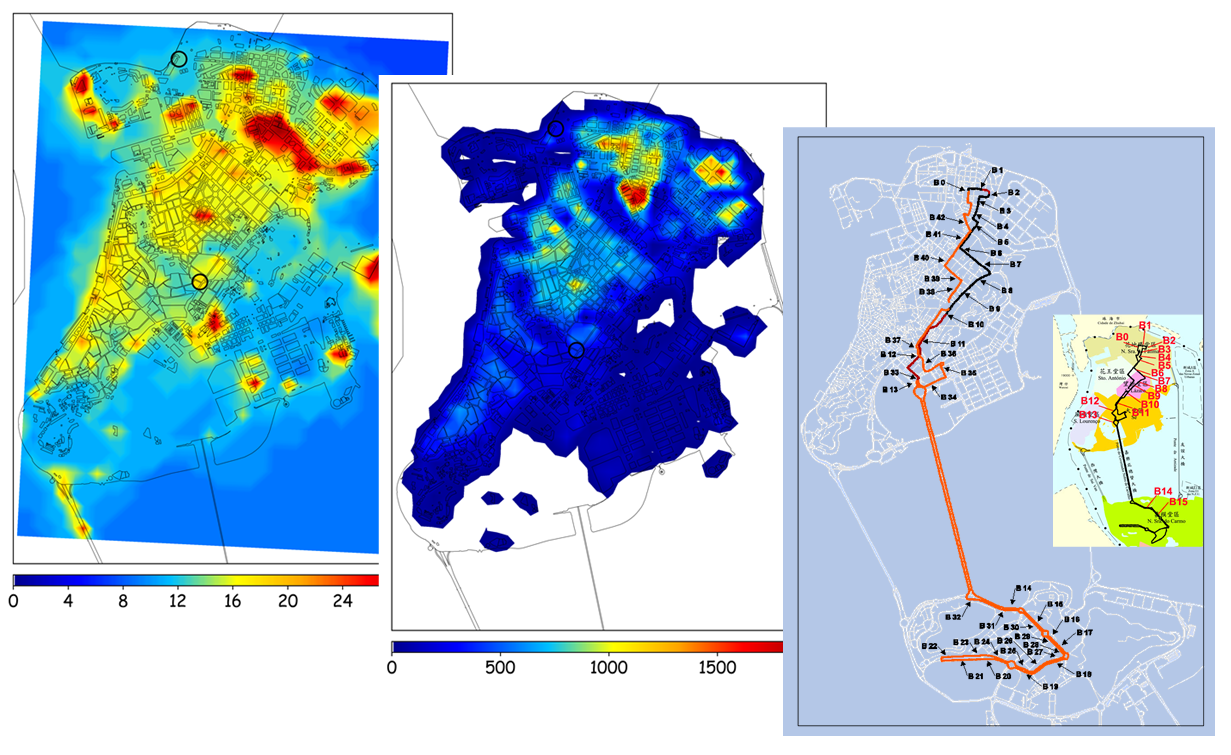
To strive for the continuous improvement of the urban air quality in Macau, our researches focus on two aspects, i.e., the ambient air quality forecasting and modelling. Following examples are some recent research outcomes:
Development of a statistical forecasting model for PM2.5 in Macau based on clustering of backward air mass trajectories
Macau is a small coastal city (~30.8 km2) in the southwestern side of the Greater Bay Area. Due to its small geographical area, statistical modelling techniques are more computationally efficient and require less input information for fulfilling the purpose of operational air quality forecasting. During the model development, it is convenient to reflect the influence of local emissions and dispersion on the air quality within the city. However, it is difficult to include the effects of transboundary pollution since there is limited information addressing the transporting pathways to Macau from various source regions. Simply speaking, the source of transboundary pollution to Macau is not known. Therefore, this study attempted to address this issue in the daily forecasting model of PM2.5 in Macau by using the clustered backward air mass trajectories of HYSPLIT from 2015 to 2017. The cluster based forecasting model was found to be more efficient (~11% improvement in the critical success index) in capturing the pollution episodes compared to the ordinary model.
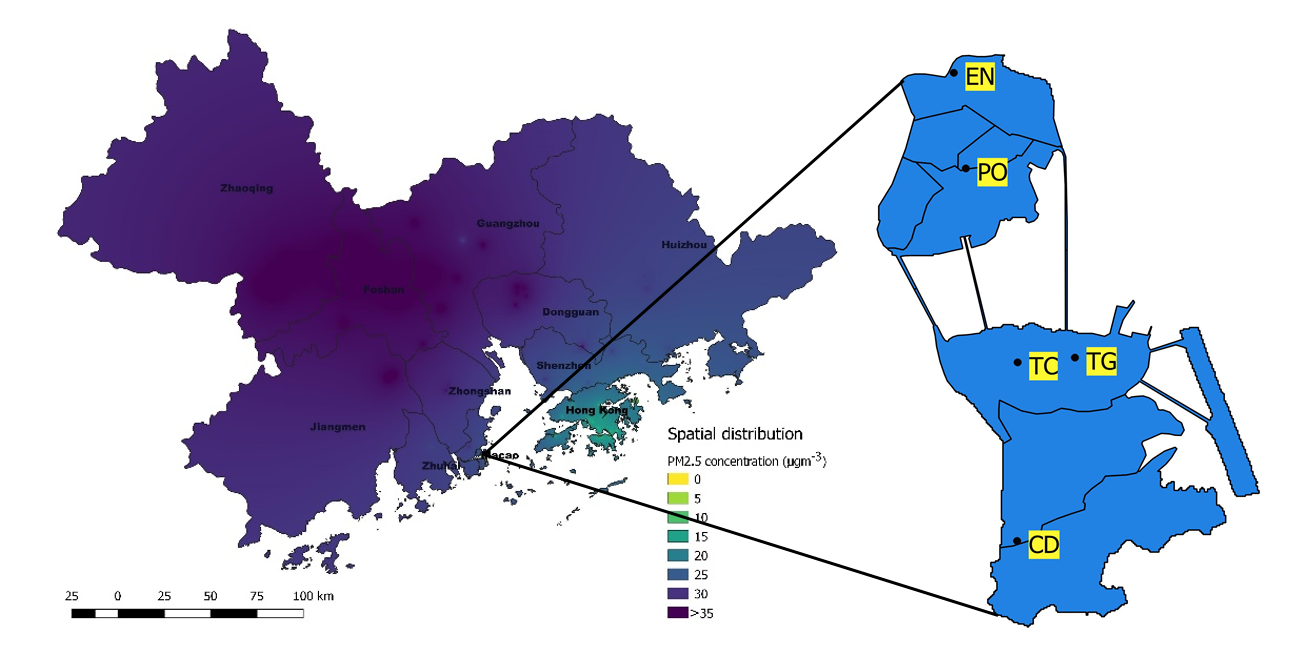
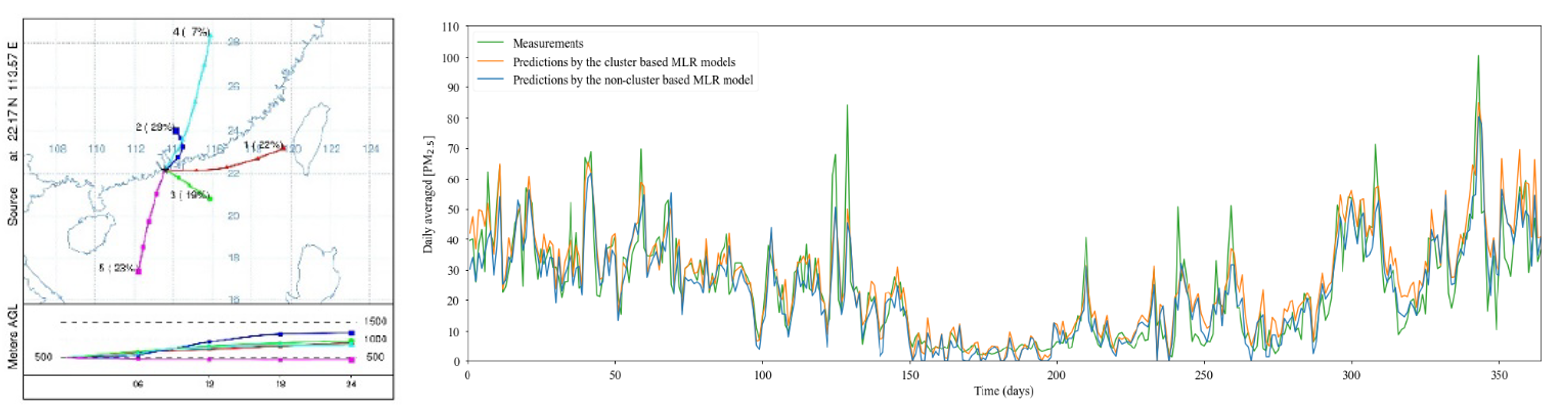
Cluster based forecasting model of PM2.5 was found to be more efficient in capturing the pollution episodes compared to the ordinary model
Predicting ground-level ozone concentrations by adaptive Bayesian model averaging of statistical seasonal models
Apart from PM2.5, the ground-level ozone is another major air pollutant of Macau or other cities in the Greater Bay Area. It is a secondary pollutant formed by the photochemical reaction of the ozone precursors (NOx, VOC). The daily variation of this pollutant has strong seasonal cycle throughout the entire year, meaning that it may not be completely described by a single model. Therefore, this study addressed the problem by proposing three ozone prediction models in different periods of the year (ozone season, non-ozone season, and the transitional period). However, sudden switching of models to their designated period in annual forecasting system may affect their performance, especially starting and ending dates of the transitional period can vary yearly. Therefore, the present study also addressed the second problem by using the adaptive Bayesian model averaging scheme. This scheme continuously evaluates the probabilities of all ozone prediction models based on the most recent ozone level. Then, the predicted ozone concentration by this scheme is obtained from the weighed sum of the model outputs according to their probabilities. Based on the measured ozone concentrations at the Taipa ambient station of the Macau Meteorological and Geophysical Bureau, results were proved to be satisfactory.
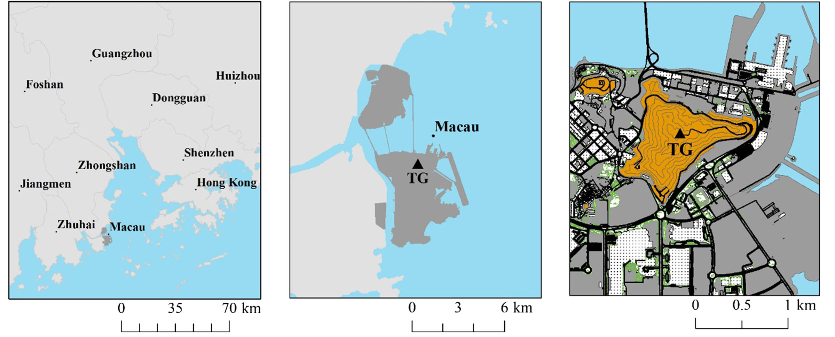
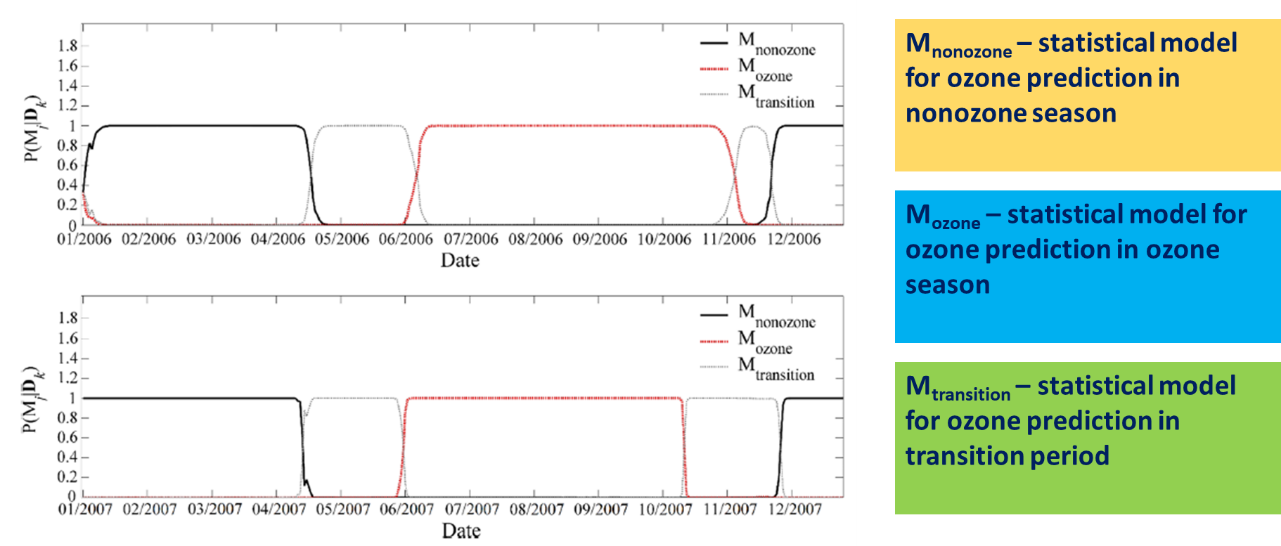
Adaptive Bayesian Model Averaging Scheme to perform averaging of ozone predictions from several models for DMA8 based on the conditional probability of each model throughout the year.
Bias correction of deterministic air quality model WRF-EURAD
The deterministic air quality model, which is the combination of the meteorological model and the chemical transport model, is widely accepted as the tool to perform operational air quality forecasting due to the large spatial coverage and its ability to explain the mechanism of the predicted pollution episodes (e.g. land-sea breeze, cold front, tropical cyclone, etc.). However, the accuracy of prediction relies on precise input information such as land cover, emission inventories, initial & boundary conditions from the global scale model, etc. Therefore, the accumulation of these uncertainties usually leads to the forecast bias. Empirical correction of the model output based on the measured pollutant concentration at the monitoring station (a.k.a. bias correction) is commonly used for improving the forecast performance. The present study attempted to develop an adaptive bias correction model by using a two-stage approach. First, a systematic selection of the most plausible bias correction model among the candidates was carried out by using the Bayesian model selection approach. Then, the Kalman filter was further implemented onto the selected model in order to transform it into an adaptive bias correction model. The two-stage approach was applied to the case study which corrects the model output of WRF-EURAD for one-day ahead prediction of PM10 concentration in Porto, Portugal. Based on the training data, the selected model at each station was found to have significantly higher probability than the other candidates, and it is also much simpler than the full model. As for the evaluation period, the corrected forecasts by the adaptive bias correction model show significant improvement over the raw outputs of WRF-EURAD. The results confirmed the success of the proposed technique.
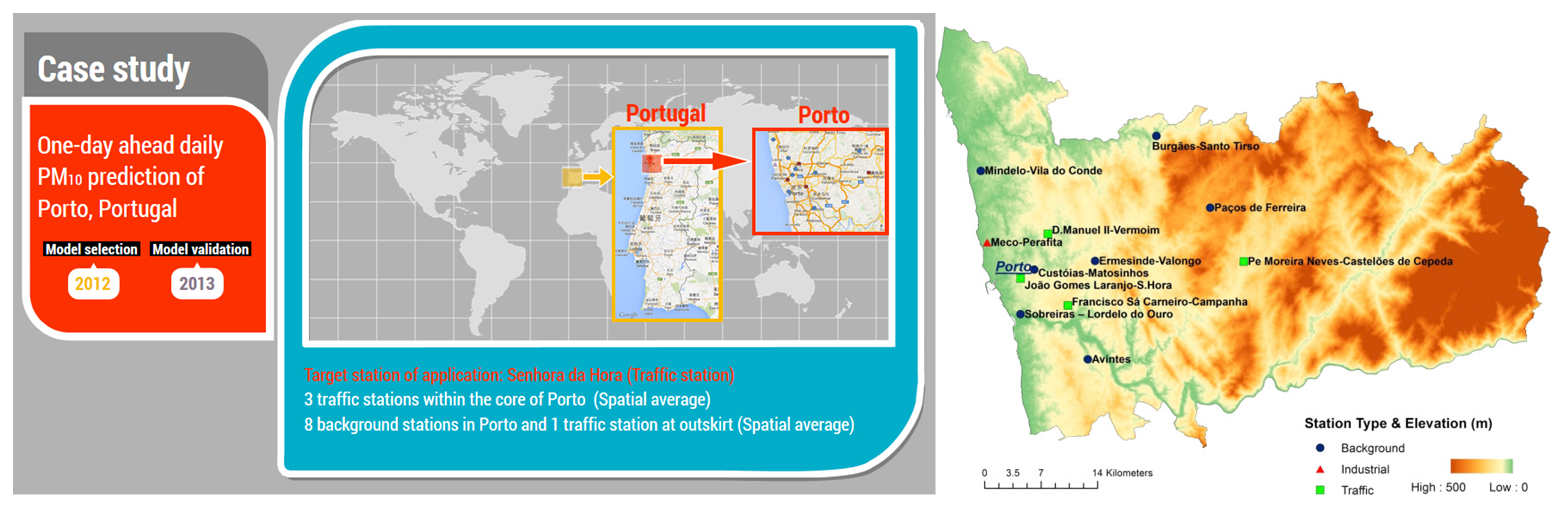
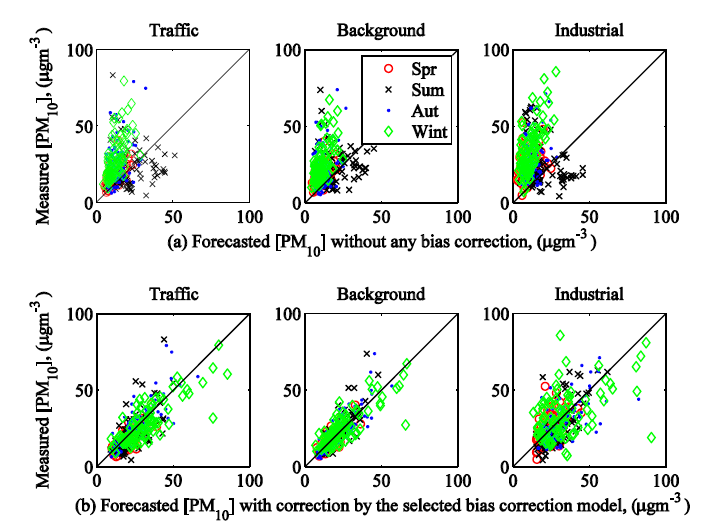
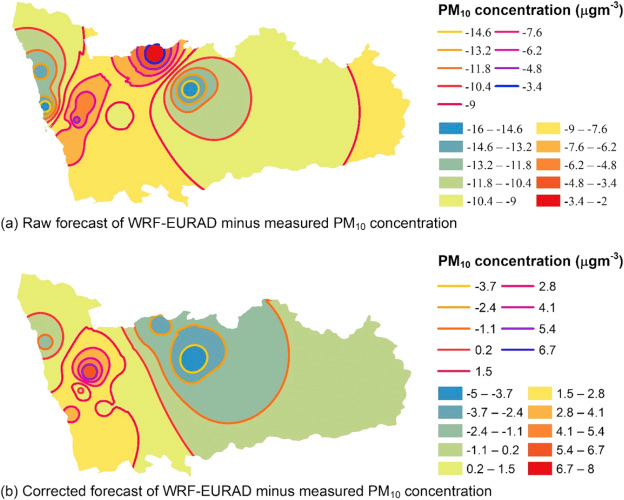
Implementation of the Kalman filter on the selected bias correction model can significantly reduce the forecast bias of PM10 concentrations in Porto, Portugal
ALL-IN-ONE Air quality modelling system applied to Macau
Air quality is one of the main environmental concerns by the Macau citizens in recent years. This region is highly affected by the pollution of respirable particulate matter (PM10 and PM2.5). The sources of PM comprise of the local anthropogenic sources (road, maritime and air transport as well as domestic, industries and construction activities) and the external influence of air pollution from its neighboring regions. Notwithstanding the potential use of air quality modelling to provide scientific advice on atmospheric emission reduction strategies, air quality forecast, air pollution assessment and air quality regulations at several spatial scales, there are only few air quality modelling applications over the Macau SAR. This study designed a multi-scale air pollution modelling system (ALL-IN-ONE) to provide scientific advice to the policy makers in their strategic planning to improve the air quality and to assess the PM pollution phenomena over Macau. The ALL-IN-ONE is based on the selection of a set of models able to reproduce the meteorological and air quality patterns, from regional to local scales. The system revealed a good performance for meteorological and air quality modelling simulations. From the modelling results, it was found that Macau was affected by air pollution transport from the neighboring regions and population was exposed to the high PM levels over entire study area. Although the tested reduction scenarios of local sources showed a reduction of PM level, several exceedances of daily concentrations were still observed. Therefore, it is necessary to join efforts with neighboring regions to discuss/apply air pollution reduction measures in order to improve the air quality of Macau. The results of this work demonstrated the importance of integrated use of modelling tools to study air pollution phenomena over Macau (ranging from regional to local scales) to define efficient air quality management strategies.
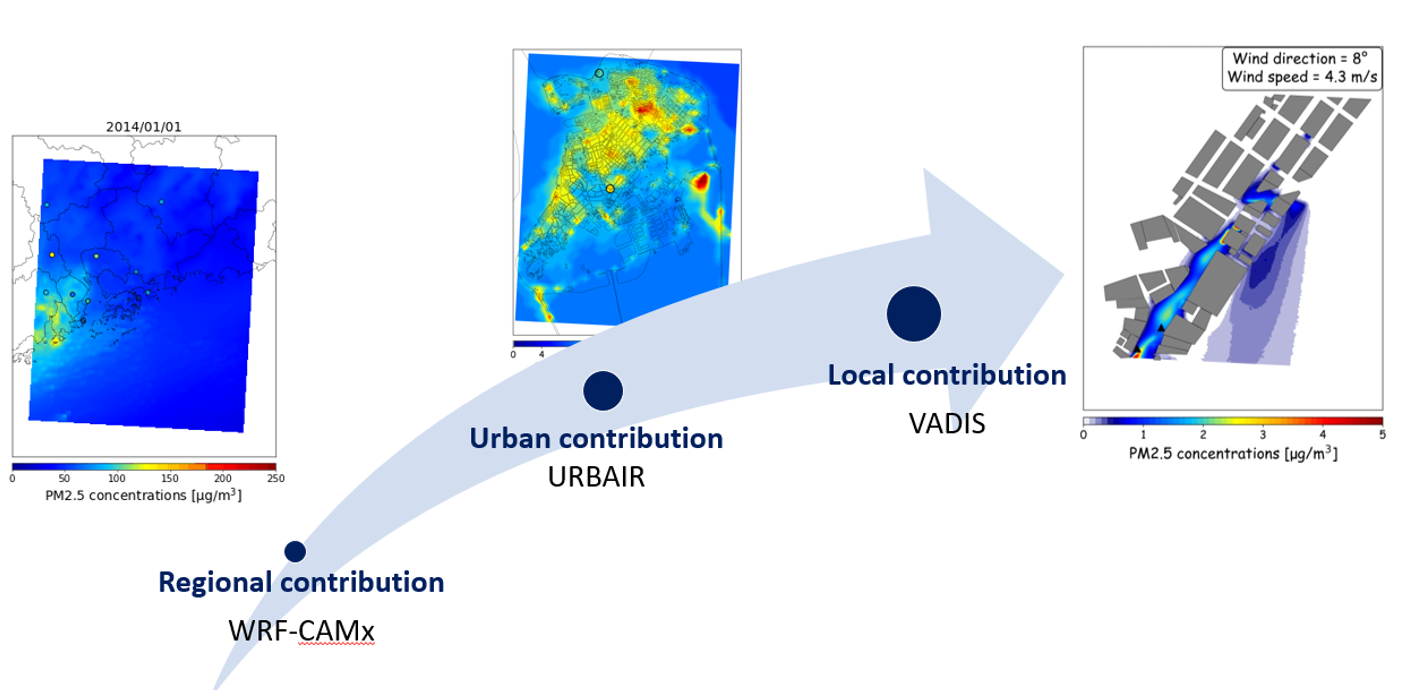
ALL-IN-ONE is an integrated modelling system addressing the contribution of air pollution of Macau from regional scale, urban scale to local scale
相關文章
- Li, X., Lopes, D., Mok, K.M., Miranda, A.I., Yuen, K.V. and Hoi K.I. (2019) Development of a road traffic emission inventory with high spatial-temporal resolution in the world’s most densely populated region-Macau, Environmental Monitoring & Assessment, Vol. 191(4), 239 | DOI: 10.1007/s10661-019-7364-9
- Lopes, D., Ferreira, J., Hoi, K.I., Miranda, A.I., Yuen, K.V. and Mok K.M. (2019) Weather Research and Forecasting Model Simulations over the Pearl River Delta Region, Air Quality, Atmosphere & Health, Vol. 12(1), 115-125.
- Liu, B., He, M.M., Wu, C., Li, J., Li, Y., Lau, N.T., Yu, J.Z., Lau, A.K.H., Fung, J.C.H., Hoi, K.I., Mok, K.M., Chan, C.K., Li, Y.J. (2019) Potential Exposure to Fine Particulate Matter (PM2.5) and Black Carbon on Jogging Trails in Macau, Atmospheric Environment, Vol. 198, 23-33.
- Mok, K.M., Yuen, K.V., Hoi, K.I., Chao, K.M. and Lopes, D. (2018) Predicting Ground-level Ozone Concentrations by Adaptive Bayesian Model Averaging of Statistical Seasonal Models, Stochastic Environmental Research and Risk Assessment, Vol. 32(5), 1283–1297.
- Hoi K. I., Mok, K. M., Miranda, A.I., Yuen, K. V., Monteiro, A., Ribeiro, I., and Lopes, D. (2018). 利用簡約函數形式對數值空氣品質預報系統進行自我調整偏差修正, 兩岸四地環境科技創新與合作青年學者論壇, August 4, Hefei, China.
- Lopes, D., Ferreira, J., Yuen, K.V., Borrego, C., Hoi, K.I., Miranda, A.I. and Mok, K.M. (2018) Desenvolvimento de um Sistema Multi-Escala de Modelação da Qualidade do ar sobre a Região Administrativa Especial de Macau, CIALP – 1º Conferência Internacional de Ambiente em Língua Portuguesa, 8-10 May, Aveiro, Portugal.
- Liu, B., He, M.M., Hoi, K.I., Mok, K.M. and Li, Y.J. (2017) Potential Exposure to Fine Particulate Matter (PM2.5) and Black Carbon on Jogging Trails in Macau, Proceedings of the 5th International Symposium on Regional Air Quality Management in Rapidly Developing Economic Regions, 16-19 November, Guangzhou, China.
- Mok, K.M., Miranda, A.I., Yuen, K.V., Hoi, K.I., Monteiro, A. and Ribeiro, I. (2017) Selection of Bias Correction Models for Improving the Daily PM10 Forecasts of WRF-EURAD in Porto, Portugal, Atmospheric Pollution Research, Vol. 8(4), 628-639.
- Hoi, K.I., Chio, C.W., Lopes D., and Mok, K.M. (2017) Seasonal Difference of PM10 Exposure in a Diesel Bus, The 5th International Symposium on Regional Air Quality Management in Rapidly Developing Economic Regions, 16-19 November, Guangzhou, China.
- Lopes, D., Hoi, K.I., Mok, K. M., Miranda, A.I., Yuen, K. V. and Borrego, C. (2016) Air Quality in the Main Cities of the Pearl River Delta Region, Global NEST Journal, Vol. 18, no.4, 794 – 802.
- Hoi, K. I., Yuen, K. V., Mok, K. M., Miranda, A.I. and Ribeiro, I. (2016), Comparison of the Offline and the Online Bias Correction of the WRF-EURAD in Porto, Portugal. In: S. Sauvage, J.M. Sánchez-Pérez & A.E. Rizzoli, (Eds.), Proceedings of the 8th International Congress on Environmental Modelling and Software, July 10-14, Toulouse, France, Vol. 2, 377 – 383.
- Lopes, D., Ferreira, J. Hoi, K. I., Mok, K. M., Miranda, A. I. and Yuen, K. V. (2016), WRF-CAMx Application to the Pearl River Delta Region, Proceedings of the 17th International Conference on Harmonisation within Atmospheric Dispersion Modelling for Regulatory Purposes (HARMO 17), May 9-12, Budapest, Hungary, 121-125.
- Lopes D., Hoi K.I., Mok K.M., Miranda A.I., Yuen K.V., Borrego C. (2015). State of the Air Quality in the Main Regions of Pearl River Delta. Proceedings of the 14th International Conference on Environmental Science and Technology, September 3-5, Rhodes, Greece, 5pp.
- Mok, K. M., Hoi, K. I., Yuen, K. V., Miranda, A. I., Borrego, C. and Ribeiro, I. (2015) Selection of a Parsimonious Empirical Correction Rule for a Deterministic Air Quality Model, 2015 International Conference on Earth Observations & Societal Impacts and ICLEI Resilience Forum, June 28 – 30, Kaohsiung, Taiwan. (Session Keynote)
- Hoi, K.I., Zhang, D.B., Mok, K.M.and Yuen, K.V. (2014) Association of Human Mortality with Air Pollution of Hong Kong, Toxics, Vol. 2, 158 – 164.
- Hoi, K. I., Yuen, K. V., Mok, K. M., Miranda, A. I. and Lai, K. U.(2014) Selection of the Daily PM10 Forecasting Model for an Urban Area in the Northern Region of Portugal with the Bayesian Approach, 2014 International Aerosol Conference, Aug. 28 – Sept. 2, Busan, Korea.
- Hoi, K.I., Yuen, K.V. and Mok, K. M. (2013) Improvement of the Multilayer Perceptron for Air Quality Modelling through an Adaptive Learning Scheme, Computers & Geosciences, Vol. 59, 148 – 155.
- Hoi, K. I., Mok, K. M., Yuen, K. V. and Pun, M. H. (2013) Investigation of Fine Particulate Pollution in a Coastal City with a Mobile Monitoring Platform, Global NEST Journal, Vol. 15, no.2, 178 – 187.
- Hoi, K. I., Yuen, K. V. and Mok, K. M. (2013) Bayesian Model Class Selection of Daily Ground-level Ozone Prediction Model, Proceedings of the 4th International Conference on Environmental Management, Engineering, Planning and Economics (CEMEPE) and SECOTOX Conference, June 24-28, Mykonos Island, Greece, 425 – 431.
- Chao, K.M., Hoi, K.I., Yuen, K.V. and Mok, K.M. (2012) Adaptive Modelling of the Daily Behavior of the Boundary Layer Ozone in Macau. ISRN Meteorology, Vol. 2012, Article ID 434176, 7 pages. doi:10.5402/2012/434176.
- Hoi, K. I., Yuen, K. V., and Mok, K. M. (2011) Iterative Probabilistic Approach for Selection of Time-varying Model Classes, Procedia Engineering, 14, 2585 – 2592.
- Hoi, K. I., Mok, K. M., Yuen, K. V. and Pun, M. H. (2011) Assessment of Fine Particulate Pollution in Macau Using a Mobile Monitoring Platform, Proceedings of the 12th International Conference for Environmental Science and Technology, September 8-10, 2011, Rhodes Island, Greece, A, 737-744.
- Hoi, K.I., Yuen, K.V. and Mok, K.M. (2010) Optimizing the Performance of Kalman Filter Based Statistical Time-varying Air Quality Models. Global NEST Journal, Vol. 12, no.1, 27 – 39.
- Hoi, K.I., Mok, K.M., Yuen, K.V., Pun, M.H., Cheng, A.Y.S., Visue, A. and Vong M.H. (2010) Spatial and temporal variation of black carbon pollution in a coastal city accessed using a mobile monitoring platform, Urban Environmental Pollution: Overcoming Obstacles to Sustainability and Quality of Life (UEP2010), 20-23 June 2010, Boston, USA, P1.20.
- Pun, M.H., Hoi, K.I., Mok, K.M., Yuen, K.V., Cheng, A.Y.S., Visue, A. and Vong M.H. (2010) Transforming the WPS measurements from a mobile monitoring platform into PM10 mass concentrations for city air quality assessment, Urban Environmental Pollution: Overcoming Obstacles to Sustainability and Quality of Life (UEP2010), 20-23 June 2010, Boston, USA P1.38.
- Hoi, K. I., Yuen, K. V., and Mok, K. M. (2010) Is a Complex Neural Network Based Air Quality Prediction Model Better Than a Simple One? A Bayesian Point of View, Proceedings of the 2nd International Symposium on Computational Mechanics in conjunction with the 12th International Conference on the Enhancement and Promotion of Computational Methods in Engineering and Science, Ed. by Lu, J.W.Z., Leung, A.Y.T., Iu, V.P. & Mok, K.M., American Institute of Physics, Melville, NY, Part I, 764 – 769.
- Hoi, K. I., Yuen, K. V. and Mok, K. M. (2009) Prediction of Daily Averaged PM10 Concentrations by Statistical Time-varying Model, Atmospheric Environment, 43, issue 16, 2579-2581.
- Hoi, K. I., Yuen, K. V. and Mok, K. M. (2009) Enhancement of Kalman Filter Based Air Quality Predictive System by Using Bayesian Approach, Proceedings of the 11th International Conference for Environmental Science and Technology, September 3-5, 2009, Chania, Crete, Greece, A, 457-466.
- Mok, K. M., Miranda, A. I., Leong, K. U and Borrego, C. (2008) A Gaussian Puff Model with Optimal Interpolation for Air Pollution Modelling, International Journal of Environment and Pollution, Vol. 35, no. 1, 111–137.
- Hoi, K. I., Yuen, K. V. and Mok, K. M. (2008) Kalman Filter Based Prediction System for Wintertime PM10 Concentrations in Macau, Global NEST Journal, 10, no.2, 140–150.
- Hoi, K. I., Yuen, K. V. and Mok, K. M. (2008) Time-varying Models for Forecasting Summertime Daily Averaged PM10 Concentrations of Macau, Proceedings of the1st Nanshan District Academic Forum of PhD Candidates’ from Shenzhen, Hong Kong, Macao and Taiwan in conjunction with the196th Tsinghua Forum for Doctoral Candidates, November 28-29, 2008,Shenzhen, China, 10pp. (Second Prize of the Best Paper Award)
- Hoi, K. I., Yuen, K. V. and Mok, K. M. (2008) An Artificial Neural Network Model for the Prediction of Daily Averaged PM10 Concentrations in Macau, Proceedings of the 6th National Civil Engineering Forum for Graduate Students, November 22-23, 2008, Beijing, China.
- Hoi, K. I., Yuen, K. V. and Mok, K. M. (2007) Statistical Modeling of PM10 concentrations in Macau by using Kalman filter, Proceedings of the APCOM’07 in conjunction with EPMESC XI (CD-ROM), December 3-6, 2007, Kyoto, Japan, MS41-4-4, 9pp.
- Hoi, K. I., Yuen, K. V. and Mok, K. M. (2007) Applying Kalman Filter to Forecast Short-term Air Quality in Macau, Proceedings of the 10th International Conference for Environmental Science and Technology, September 5-7, 2007, COS Island, Greece, Vol. A, 504-511.
- Chang, S. W., Mok, K. M. and Yuen, K. V. (2007) Association of PM10 Pollution Episodes with the Meterological Conditions in Macau, Proceedings of the 10th International Conference for Environmental Science and Technology, September 5-7, 2007, COS Island, Greece, Vol. B, 90-95.
- Yuen, K. V., Hoi K. I., and Mok, K. M. (2007) On the Selection of Noise Parameters for Kalman Filter, Journal of Earthquake Engineering and Engineering Vibration, Vol. 6, no. 1, 49 – 56.
- Hoi K. I., Mok K. M., and Yuen K.-V. (2006) Analysis of Wet Acid Deposition in Macau, Global NEST Journal, Vol. 8, no.3, 224 – 233.
- Hoi K. I., Yuen, K. V. and Mok, K. M. (2006) Updating Noise Parameters of Kalman Filter Using Bayesian Approach, Computational Methods in Engineering & Science: Proceedings of the EPMESC X, by Yao, Z.H., Yuan, M.W. & Chen, Y.Q., Tsinghua University Press & Springer, Beijing, 782 – 791. (EPMESC X Student Paper Competition Award)
- Lam, L. H. and Mok, K. M. (2006) Prediction of PM10 Concentrations in Macao with Artificial Neural Network, Computational Methods in Engineering & Science: Proceedings of the EPMESC X, Ed. by Yao, Z.H., Yuan, M.W. & Chen, Y.Q., Tsinghua University Press & Springer, Beijing, 895 – 902.
- Mok, K.M. , Hoi, K.I. and Yuen K.V. (2005) Causes of Wet Acid Deposition in Macau, Proceedings of the 9th International Conference on Environmental Science and Technology, September 1 – 3, 2005, Rhodes island, Greece, Vol. B, 623-628.
- Mok, K. M. and Hoi, K. I. (2005) Effects of Meteorological Conditions on PM10 Concentrations – A Study in Macau, Environmental Monitoring and Assessment, Vol. 102, 201-223.
- Tsai, W. Y., Chan, L. Y., Chan, C. Y., Wong, K. H. and Mok, K. M. (2004) Respirable Suspended Particulate in Macao, The 3rd Asian Aerosol Conference, January 6 – 9, 2004, Hong Kong, (Fri-B2, OAA05).
- Mok, K. M. and Hoi, K. I. (2003) Association of PM10 Concentrations and Wind Directions in Macau, Proceedings of the International Conference on Pollution in Metropolitan and Urban Environment – POLMET 2003 (POLMET CD Rom), Nov. 3-5, 2003, Hong Kong, Session 6A, 6pages.
- Chan, L. Y., Wong, K. H., Chan, C. Y., Tsai, W. Y. and Mok, K. M. (2002) Carbon Monoxide in Urban Roadside Microenvironments of Macao, Proceedings of the Regional Workshop on Better Air Quality in Asian and Pacific Rim Cities (BAQ 2002 CD Rom), Hong Kong, PS-34-1 – PS-34-4.
- Mok, K. M. (2002) Development of an Air-Quality Index Forecasting System for Macau – A Memoir, Investigação Científica Sobre o Ambiente e Desenvolvimento Urbano em Macau, Ed. by Wang, Z.S., Chen, J.N., & Du, P.F., Univ. of Macau, Tsinghua Univ. & National Natural Science Foundation, Macau, 34-46. (Invited Lecture)
- Mok, K. M., Tam, S. C., Hoi, K. I. and Lei, S. I. (2002) Um Sistema “Inteligente” de Previsão da Qualidade do ar em Macau, Revista do Ambiente, ANO 6, No. 20-21, 92-96.
- Mok, K. M., Tam, S. C., Hoi, K. I. and Lei, S. I. (2002) An Intelligent Air-Quality Forecasting System in Macao, Proceedings of the 2001 International Conference and Exhibition on Sustainable Development and Green Enterprises, Ed. By Environment Council, Macao Special Administrative Region, P.R. China, Environment Council, 273-282. (Invited Lecture)
- Mok, K. M., Tam, S. C., Yan, P. and Lam, L. H. (2000) A Neural Network Forecasting System for Daily Air Quality Index in Macau, Air Pollution VIII, Ed. by Longhurst, J.W.S., Brebbia, C.A., & Power, H., WIT Press, Southampton, 41-50.
- Mok, K. M., Tam, S. C. and Wang, Z. S. (1999) Air-Quality Forecasting System in Macau, Proceedings of the 1st Macau Symposium on Environment and City Development, Macau, 78-85.
- Mok, K. M., Tam, S. C., Lam, L. H., and Yan, P. (1999) Prediction of Macau Daily Electricity Consumption with Artificial Neural Network, Computational Methods in Engineering and Science – EPMESC VII, Ed. by Bento, J., Oliveira, E. A. & Pereira, E., Elsevier Science, Kidlington, Oxford, Vol. 2, 827 – 836.
- Tam, S. C., Mok, K. M., Yan, P., and Lam, L. H. (1999) An Adaptive Neuro-Fuzzy Inference System for the Forecast of Macau Daily Electric Load, Computational Methods in Engineering and Science – EPMESC VII, Ed. by Bento, J., Oliveira, E. A. & Pereira, E., Elsevier Science, Kidlington, Oxford, Vol. 2, 817 – 826.
- Mok, K. M. (1998) INTELAIR-Hybrid System for Urban Air Pollution Management: Artificially Intelligent and Traditional Modelling, Proceedings of S&T Co-Operation with Asia in the Area of Sustainable Management of Natural Resource – A Co-Ordination Meeting in China (CD-ROM), Ed. by J. P. Lobo-Ferreira and Tilak Viegas, CEC-DGXII-INCO-DC and P.R. China-SSTC-DIC. (Invited Presentation)
- Mok, K. M. and Tam, S. C., (1998) Short-Term Prediction of SO2 Concentration in Macau with Artificial Neural Networks, Energy and Buildings, Vol. 28, 279-286.
- Tam, S. C., Mok, K. M. and Sam, C. (1998) Adaptive Fuzzy System for Prediction of SO2 concentration in Macau, Air Pollution VI, Ed. by Brebbia, C.A., Ratto, C.F. & Power, H., WIT Press/Computational Mechanics Publications, Southampton, 277-286.